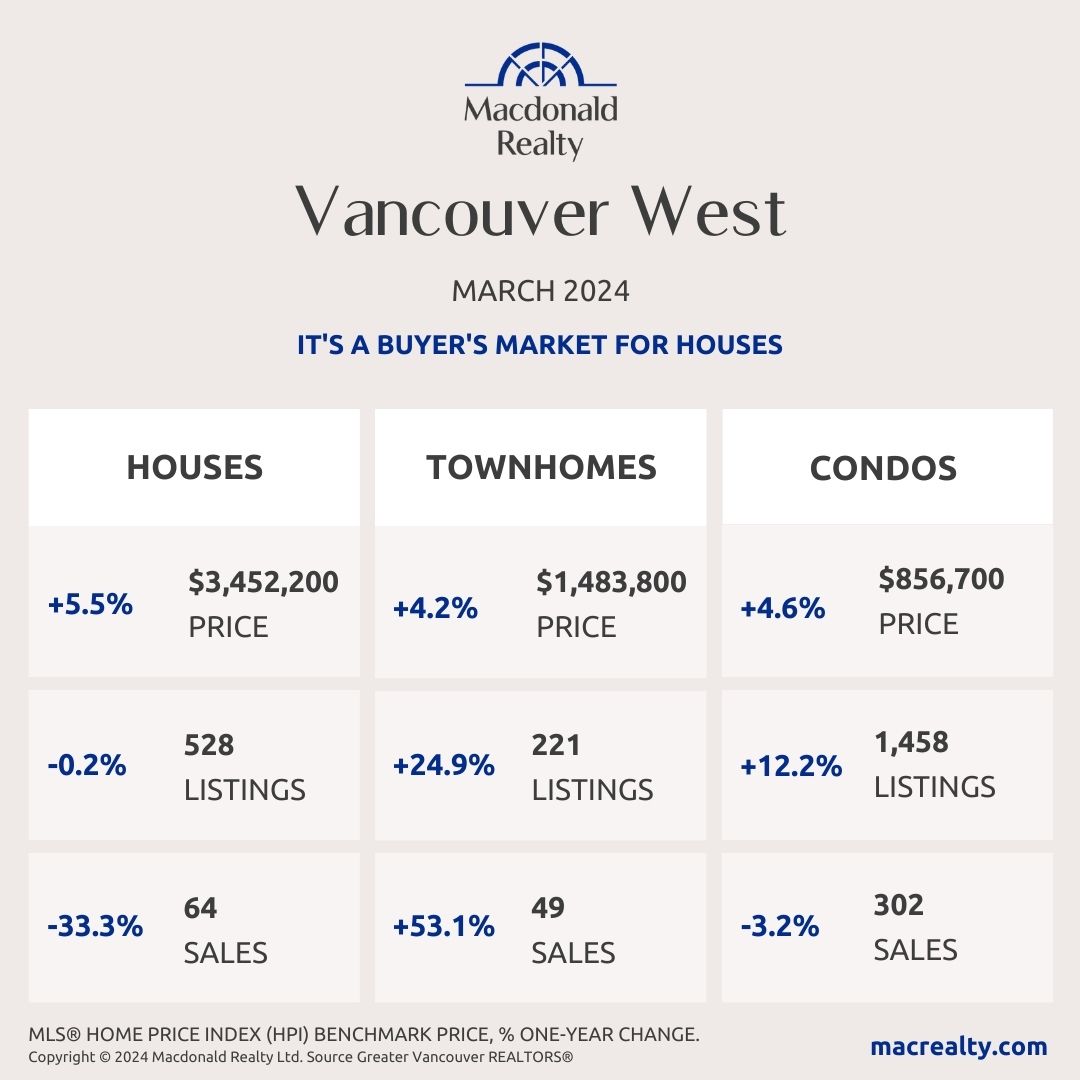
How to Calculate b0 and b1 in Linear Regression: A Clear Guide
Linear regression is a statistical technique used to model the relationship between two variables, where one variable is considered to be the predictor or independent variable, and the other variable is considered to be the response or dependent variable. The goal of linear regression is to find the best-fit line that describes the relationship between the two variables. The line is described by an equation of the form y = b0 + b1x, where b0 is the intercept and b1 is the slope of the line.
Calculating b0 and b1 is an essential part of the linear regression process. The intercept, b0, represents the point where the regression line intersects the y-axis, and the slope, b1, represents the change in the response variable for a one-unit change in the predictor variable. These coefficients are used to make predictions about the response variable based on the values of the predictor variable.
There are several methods for calculating b0 and b1, including the least squares method, which is the most commonly used method. The least squares method involves minimizing the sum of the squared differences between the observed values of the response variable and the predicted values based on the regression line. By finding the values of b0 and b1 that minimize this sum, we can find the best-fit line that describes the relationship between the two variables.
Understanding Linear Regression
Definition and Use Cases
Linear regression is a statistical method used to establish a relationship between a dependent variable and one or more independent variables. The goal is to predict the value of the dependent variable based on the value of the independent variable(s). Linear regression is used extensively in many fields including finance, economics, social sciences, engineering, and more.
Linear regression can be classified into two types: simple linear regression and multiple linear regression. Simple linear regression involves only one independent variable, while multiple linear regression involves two or more independent variables.
Simple linear regression is commonly used in scenarios where the relationship between two variables is linear. For example, in finance, simple linear regression can be used to predict the stock price of a company based on its earnings per share. In social sciences, it can be used to predict the relationship between two variables such as income and happiness.
Assumptions in Linear Regression
Linear regression relies on several assumptions to make accurate predictions. These assumptions include:
- Linearity: The relationship between the dependent variable and independent variable(s) is linear.
- Independence: The observations are independent of each other.
- Homoscedasticity: The variance of the errors is constant across all levels of the independent variable(s).
- Normality: The errors are normally distributed.
If these assumptions are not met, the predictions made by linear regression may not be accurate. Therefore, it is important to check these assumptions before using linear regression to make predictions.
Fundamentals of Linear Regression Equations
Equation Overview
Linear regression is a statistical method used to model the relationship between two variables. In simple linear regression, one variable is considered as the independent variable, while the other variable is considered as the dependent variable. The goal of linear regression is to find the best-fitting straight line that describes the relationship between the two variables.
The equation for a straight line is y = mx + b, where y is the dependent variable, x is the independent variable, m is the slope of the line, and b is the y-intercept. In linear regression, the slope and y-intercept are estimated from the data using the least squares method.
The equation for a simple linear regression model is y = b0 + b1x, where y is the dependent variable, x is the independent variable, b0 is the y-intercept, and b1 is the slope of the line. The goal of linear regression is to estimate the values of b0 and b1 that best fit the data.
Role of b0 and b1
In the simple linear regression equation y = b0 + b1x, b0 is the y-intercept, which represents the predicted value of y when x = 0. The value of b1 is the slope of the line, which represents the change in y for a unit change in x.
The slope of the line is an important parameter in linear regression because it indicates the direction and strength of the relationship between the two variables. If b1 is positive, then there is a positive relationship between x and y, meaning that as x increases, y also increases. If b1 is negative, then there is a negative relationship between x and y, meaning that as x increases, y decreases.
The y-intercept is also an important parameter in linear regression because it represents the predicted value of y when x = 0. This value can be interpreted as the baseline value of y, or the value of y when x has no effect on it.
In summary, the simple linear regression equation y = b0 + b1x is used to model the relationship between two variables. The values of b0 and b1 are estimated from the data using the least squares method, and they represent the y-intercept and slope of the line, respectively.
Calculating b1: Slope of the Line
Formula for b1
The slope of the line, denoted by b1, represents the change in the response variable (y) per unit change in the predictor variable (x). The formula for b1 is:
b1 = Σ[(xi - x̄)(yi - ȳ)] / Σ[(xi - x̄)2]
where xi is the ith value of the predictor variable, x̄ is the mean of the predictor variable, yi is the ith value of the response variable, and ȳ is the mean of the response variable.
Step-by-Step Calculation
To calculate b1, the following steps can be followed:
- Calculate the mean of the predictor variable (x̄) and the mean of the response variable (ȳ).
- For each observation, calculate (xi - x̄) and (yi - ȳ).
- Multiply (xi - x̄) and (yi - ȳ) for each observation to get [(xi - x̄)(yi - ȳ)].
- Sum up all [(xi - x̄)(yi - ȳ)] from step 3 to get Σ[(xi - x̄)(yi - ȳ)].
- For each observation, calculate (xi - x̄)2.
- Sum up all (xi - x̄)2 from step 5 to get Σ[(xi - x̄)2].
- Divide Σ[(xi - x̄)(yi - ȳ)] by Σ[(xi - x̄)2] to get b1.
Interpreting b1
The value of b1 indicates the direction and strength of the relationship between the predictor and response variables. If b1 is positive, it means that an increase in the predictor variable is associated with an increase in the response variable. If b1 is negative, it means that an increase in the predictor variable is associated with a decrease in the response variable. The magnitude of b1 indicates the strength of the relationship, with larger values indicating a stronger relationship.
Calculating b0: Y-Intercept
Formula for b0
The y-intercept (b0) is the point where the regression line crosses the y-axis. It represents the predicted value of y when x is equal to 0. The formula for b0 is:
b0 = ȳ - b1x̄
Where:
- ȳ is the mean of the dependent variable y
- x̄ is the mean of the independent variable x
- b1 is the slope of the regression line
Step-by-Step Calculation
To calculate b0, follow these steps:
- Calculate the mean of the dependent variable y (ȳ).
- Calculate the mean of the independent variable x (x̄).
- Calculate the slope of the regression line (b1).
- Plug the values of ȳ, x̄, and b1 into the formula for b0.
For example, let's say you have the following data:
x | y |
---|---|
1 | 3 |
2 | 5 |
3 | 7 |
4 | 9 |
5 | 11 |
Calculate the mean of y:
ȳ = (3 + 5 + 7 + 9 + 11) / 5 = 7
Calculate the mean of x:
x̄ = (1 + 2 + 3 + 4 + 5) / 5 = 3
Calculate the slope of the regression line:
b1 = Σ[(xi - x̄)(yi - ȳ)] / Σ(xi - x̄)2 = 2
Plug the values of ȳ, x̄, and b1 into the formula for b0:
b0 = 7 - 2(3) = 1
Therefore, the equation of the regression line is:
ŷ = 1 + 2x
Interpreting b0
Interpreting b0 is straightforward. It represents the predicted value of y when x is equal to 0. In other words, it is the y-intercept of the regression line. For example, if b0 is equal to 1, it means that the predicted value of y when x is equal to 0 is 1.
It is important to note that the interpretation of b0 depends on the context of the problem. For example, if the independent variable x represents time, and the dependent variable y represents the price of a stock, then b0 represents the predicted price of the stock at the beginning of the time period.
Using Statistical Software
Software Options
There are many statistical software options available to calculate b0 and b1 in linear regression. Some popular options include R, SAS, mortgage payment calculator massachusetts SPSS, and Stata. Each software has its own strengths and weaknesses, so it is important to choose the one that best fits your needs.
Inputting Data
To calculate b0 and b1 in linear regression using statistical software, you will need to input your data into the software. This can typically be done by importing a data file or manually entering the data into the software.
It is important to ensure that your data is in the correct format before inputting it into the software. This may include formatting variables as numeric or categorical, checking for missing data, and ensuring that your data is in the correct order.
Interpreting Output
Once you have inputted your data into the software and run the linear regression analysis, you will need to interpret the output. This typically includes examining the coefficients for b0 and b1, as well as the p-values and confidence intervals for each coefficient.
It is important to note that the interpretation of the output may vary depending on the software used. However, a general understanding of the output and its interpretation can be useful in understanding the results of your analysis.
Overall, using statistical software to calculate b0 and b1 in linear regression can be a powerful tool for data analysis. By choosing the right software, inputting your data correctly, and interpreting the output, you can gain valuable insights into your data and make informed decisions based on your findings.
Interpreting and Validating Results
Coefficient of Determination (R^2)
The coefficient of determination, or R^2, measures the proportion of the variance in the dependent variable that is predictable from the independent variable. It ranges from 0 to 1, with higher values indicating a better fit of the regression line to the data.
An R^2 value of 1 indicates that the regression line perfectly fits the data, while an R^2 value of 0 indicates that the regression line does not fit the data at all. However, it is important to note that a high R^2 value does not necessarily mean that the regression model is a good predictor of the dependent variable, as there may be other factors that influence the relationship between the independent and dependent variables.
Residual Analysis
Residual analysis is a method used to validate the assumptions of linear regression and check for the presence of outliers or influential points. Residuals are the differences between the observed values and the predicted values of the dependent variable.
A residual plot can be used to visually inspect the residuals and check for patterns or trends. Ideally, the residuals should be randomly scattered around zero, indicating that the regression model is a good fit for the data. However, if there is a pattern or trend in the residuals, it may indicate that the regression model is not a good fit for the data.
Another method of residual analysis is to check for outliers or influential points. An outlier is an observation that is significantly different from the other observations in the data set, while an influential point is an observation that significantly affects the regression line. These points can have a large impact on the regression model and should be carefully examined to determine whether they should be included or excluded from the analysis.
Overall, interpreting and validating the results of linear regression is an important step in determining the accuracy and reliability of the model. By examining the coefficient of determination and conducting residual analysis, researchers can gain insight into the relationship between the independent and dependent variables and identify any potential issues with the regression model.
Practical Considerations
Data Quality and Preparation
Before performing linear regression analysis, it is important to ensure that the data is of high quality and that it has been prepared appropriately. This includes checking for missing values, outliers, and ensuring that the data is normally distributed.
If there are missing values, they can be imputed using various techniques such as mean imputation or regression imputation. Outliers can be detected using box plots or scatter plots and can be handled by removing them or transforming the data. Normality can be checked using a histogram or a normal probability plot.
It is also important to ensure that the data is scaled appropriately. If the data is not scaled, the regression coefficients may not be meaningful. Standardization or normalization can be used to scale the data.
Limitations of b0 and b1
It is important to note that b0 and b1 are estimates of the true population regression coefficients and are subject to sampling variability. Therefore, the estimates may not be accurate if the sample size is small or if there is a large amount of noise in the data.
Additionally, b0 and b1 only capture the linear relationship between the predictor variable and the response variable. If there is a non-linear relationship, such as a quadratic or exponential relationship, a linear regression model may not be appropriate.
Finally, it is important to note that correlation does not imply causation. Just because there is a significant relationship between the predictor variable and the response variable, it does not necessarily mean that one causes the other. Other factors may be involved and should be considered when interpreting the results.
Frequently Asked Questions
What is the process for computing the y-intercept (b0) in linear regression?
The y-intercept (b0) in linear regression represents the predicted value of the dependent variable when the independent variable is zero. To compute b0, the regression equation is run with the independent variable set to zero. The resulting value is the y-intercept.
How is the slope (b1) determined in a linear regression model?
The slope (b1) in a linear regression model represents the change in the dependent variable for every one-unit change in the independent variable. To determine b1, the regression model is run, and the resulting slope is calculated using the formula: b1 = (nΣXY - ΣXΣY) / (nΣX^2 - (ΣX)^2), where n is the number of observations, X is the independent variable, Y is the dependent variable, Σ is the sum of, and ^2 represents squared.
What steps are involved in interpreting the coefficient b1 in a regression analysis?
Interpreting the coefficient b1 in a regression analysis involves understanding the direction and magnitude of the relationship between the independent and dependent variables. A positive b1 indicates a positive relationship, while a negative b1 indicates a negative relationship. The magnitude of b1 represents the strength of the relationship. A larger absolute value of b1 indicates a stronger relationship between the variables.
In the context of regression analysis, what does b0 represent?
In the context of regression analysis, b0 represents the y-intercept of the regression line. It is the predicted value of the dependent variable when the independent variable is zero.
Can you explain how to use Excel for calculating the regression coefficients b0 and b1?
To calculate the regression coefficients b0 and b1 in Excel, use the LINEST function. The LINEST function returns an array of values that includes the slope (b1) and y-intercept (b0). The formula for the LINEST function is LINEST(y-values, x-values, [const], [stats]), where y-values and x-values are the arrays of dependent and independent variables, respectively. The optional const argument is set to TRUE to indicate that the y-intercept should be calculated, and the optional stats argument is set to FALSE to indicate that additional regression statistics should not be calculated.
What is the significance of b0 and b1 in the context of a linear regression equation?
The significance of b0 and b1 in the context of a linear regression equation is that they determine the slope and intercept of the regression line, which is used to predict the value of the dependent variable based on the value of the independent variable. The slope (b1) indicates the rate of change in the dependent variable for every one-unit change in the independent variable, while the y-intercept (b0) represents the predicted value of the dependent variable when the independent variable is zero.