How to Calculate Bias: A Clear and Neutral Guide
Bias is a statistical term that refers to the systematic deviation of an estimator from the true value of a parameter. It is a common problem in many fields, including science, medicine, and finance. Bias can arise from a variety of sources, such as measurement error, sampling bias, or model misspecification. Therefore, understanding how to calculate bias is essential for anyone who wants to make accurate predictions or draw valid conclusions from data.
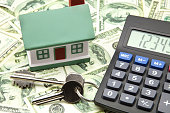
One way to calculate bias is to compare the expected value of the estimator to the true value of the parameter being estimated. If the expected value is equal to the true value, the estimator is unbiased. However, if the expected value is different from the true value, the estimator is biased. The amount of bias can be quantified by taking the difference between the expected value and the true value. A positive bias means that the estimator tends to overestimate the true value, while a negative bias means that the estimator tends to underestimate the true value.
There are many techniques for reducing bias in estimation, such as using more accurate measurement instruments, increasing the sample size, or using more flexible models. However, it is important to note that bias cannot be completely eliminated, and it is always necessary to assess the quality of the estimation method and the sources of bias. By understanding how to calculate bias and how it affects the accuracy of estimates, researchers and practitioners can make more informed decisions and avoid costly mistakes.
Understanding Bias
Definition of Bias
Bias is a systematic error in the measurement or estimation of a variable. It occurs when there is a consistent difference between the true value of the variable and the measured or estimated value. Bias can be introduced at any stage of the research process, from study design to data analysis.
In statistical terms, bias is the difference between the expected value of an estimator and the true value of the parameter being estimated. An estimator is a statistic that is used to estimate a parameter, such as the mean or variance of a population. Bias can lead to incorrect conclusions and can affect the validity of research findings.
Types of Bias
There are several types of bias that can occur in research studies. Some of the most common types of bias include:
Selection bias: This occurs when the selection of participants is not random, or when there is a systematic difference between the characteristics of those who participate and those who do not. This can lead to over- or under-representation of certain groups in the sample, which can affect the generalizability of the results.
Measurement bias: This occurs when there is a systematic error in the measurement of a variable. This can be due to faulty equipment, observer bias, or other factors that affect the accuracy of the measurement.
Reporting bias: This occurs when there is a selective reporting of results, such as when only positive results are reported or when negative results are suppressed. This can lead to an overestimation of the effect size and can affect the interpretation of the results.
Publication bias: This occurs when studies with significant results are more likely to be published than studies with non-significant results. This can lead to an overestimation of the effect size and can affect the validity of meta-analyses.
Understanding the different types of bias is important for researchers to identify potential sources of bias in their studies and to take steps to minimize or eliminate them.
Bias in Statistical Analysis
Role of Bias in Statistics
Bias is an important concept in statistical analysis as it can affect the accuracy of the results. Bias in statistics refers to a systematic error that occurs when the results of a statistical analysis are not representative of the population being studied. In other words, bias occurs when the statistical analysis is skewed in a particular direction, leading to inaccurate or misleading conclusions.
Common Biases in Statistical Models
There are many different types of biases that can occur in statistical models. Some of the most common include:
Selection Bias: This occurs when the sample used in the statistical analysis is not representative of the population being studied. For example, if a study on the effectiveness of a new drug only includes patients who are already healthy, the results may be biased as the drug may not be as effective for patients who are already sick.
Measurement Bias: This occurs when the method used to measure the variables being studied is inaccurate or biased. For example, if a study on the effectiveness of a new weight loss program only uses self-reported weight measurements, the results may be biased as participants may not report their weight accurately.
Sampling Bias: This occurs when the sample used in the statistical analysis is not random or representative of the population being studied. For example, if a study on the effectiveness of a new teaching method only includes students from one school district, the results may be biased as the teaching method may not be as effective for students from other districts.
Survivorship Bias: This occurs when the statistical analysis only includes data from subjects that have survived or succeeded in a particular area, while ignoring data from subjects that have failed or dropped out. For example, if a study on the success rates of entrepreneurs only includes successful entrepreneurs, the results may be biased as the study is not accounting for the many entrepreneurs who have failed.
It is important to be aware of these biases when conducting statistical analysis to ensure that the results are accurate and representative of the population being studied.
Calculating Bias
Formulas for Calculating Bias
Bias is an important concept in statistics, and it is essential to understand how to calculate it accurately. The formula for calculating bias depends on the type of estimator being used. If an estimator is unbiased, then its bias is equal to zero. On the other hand, if an estimator is biased, then its bias is nonzero.
The bias of an estimator H is defined as the expected value of the estimator less the value θ being estimated. Mathematically, this can be expressed as:
Bias(H) = E(H) - θ
where E(H) is the expected value of the estimator H, and θ is the true value of the parameter being estimated.
Step-by-Step Calculation Process
To calculate the bias of an estimator, you need to follow these steps:
Determine the true value of the parameter being estimated. This is usually given in the problem statement or can be estimated from the data.
Calculate the expected value of the estimator. This can be done by taking the average of the estimator over many trials or by using a theoretical formula.
Subtract the true value of the parameter from the expected value of the estimator to get the bias. If the bias is zero, then the estimator is unbiased. If the bias is nonzero, then the estimator is biased.
Interpret the bias in the context of the problem. A positive bias means that the estimator tends to overestimate the true value of the parameter, while a negative bias means that the estimator tends to underestimate the true value of the parameter.
In conclusion, calculating the bias of an estimator is an essential step in statistical analysis. By following the above steps, one can accurately calculate the bias and interpret its meaning in the context of the problem.
Bias in Machine Learning
Impact of Bias on Machine Learning
Bias in machine learning refers to the presence of systematic errors in the algorithm that can prevent it from making accurate predictions. These errors can arise from a variety of factors, such as the quality of the training data, the assumptions made by the algorithm, or the way the algorithm is designed. When bias is present, the algorithm may make predictions that are consistently off-target, leading to poor performance and incorrect decisions.
One of the most significant impacts of bias on machine learning is that it can lead to unfair or discriminatory outcomes. For example, if a machine learning algorithm is trained on data that is biased against certain groups of people, it may make predictions that systematically disadvantage those groups. This can result in discriminatory outcomes in areas such as employment, lending, and criminal justice.
Detecting and Correcting Bias in Algorithms
Detecting and correcting bias in machine learning algorithms is a critical step in ensuring that they are fair and accurate. One approach to detecting bias is to analyze the training data and look for patterns that may indicate systematic errors. For example, if the training data contains a disproportionate number of examples from one group, this may indicate bias against other groups.
Once bias has been detected, there are several approaches to correcting it. One approach is to adjust the algorithm to account for the bias, such as by weighting certain examples more heavily or adjusting the decision threshold. Another approach is to modify the training data to reduce the bias, such as by oversampling underrepresented groups or removing biased examples.
Overall, detecting and correcting bias in machine learning algorithms is an ongoing process that requires careful analysis and monitoring. By ensuring that algorithms are free from bias, we can create more accurate and fair predictions that benefit everyone.
Practical Examples
Bias Calculation in Social Sciences
In social sciences, bias can occur due to various reasons, such as non-response bias, selection bias, and measurement bias. Non-response bias occurs when some participants do not respond to the survey, resulting in a biased sample. Selection bias refers to the tendency to select participants who are not representative of the population being studied. Measurement bias occurs when the measurement tool used is not accurate or consistent.
To calculate bias in social science research, researchers can use statistical methods such as regression analysis, propensity score matching, and instrumental variable analysis. These methods can help to identify and adjust for potential sources of bias in the data.
Case Study: Bias in Healthcare Data
Bias in healthcare data can have serious consequences, such as inaccurate diagnoses and treatment recommendations. For example, a study found that a widely used algorithm for predicting healthcare needs underestimated the needs of black patients by more than twice the rate of white patients [1].
To calculate bias in healthcare data, researchers can use methods such as sensitivity analysis and subgroup analysis. Sensitivity analysis involves testing the robustness of the results by varying the assumptions or parameters used in the analysis. Subgroup analysis involves analyzing the data for specific subgroups, such as race or gender, to identify potential sources of bias.
Overall, calculating bias is an important step in ensuring the validity and reliability of research findings. By identifying and adjusting for potential sources of bias, researchers can improve the accuracy and generalizability of their results.
References
- Obermeyer, Z., Powers, B., Vogeli, C., -amp; Mullainathan, S. (2019). Dissecting racial bias in an algorithm used to manage the health of populations. Science, 366(6464), 447-453.
Mitigating Bias
Strategies to Reduce Bias
There are several strategies that can be used to mitigate bias in data and machine learning models. One strategy is to increase the diversity of the data used to train the model. This can be done by incorporating data from different sources, and by ensuring that the data is representative of the population that the model is intended to serve.
Another strategy is to use techniques such as cross-validation, bootstrapping, and regularization to reduce bias in the model. Cross-validation involves dividing the data into multiple subsets and training the model on each subset, while bootstrapping involves randomly sampling the data to create multiple training sets. Regularization involves adding a penalty term to the model's objective function to discourage overfitting.
Tools for Bias Mitigation
There are also several tools and frameworks available for mitigating bias in machine learning models. For example, the IBM AI Fairness 360 toolkit provides a set of algorithms and metrics for detecting and mitigating bias in machine learning models. The toolkit includes algorithms for debiasing data, as well as metrics for measuring bias in the model's predictions.
Another example is the Google What-If Tool, which allows users to visualize the impact of different input features on the model's predictions. The tool can be used to identify and mitigate bias in the model by exploring different scenarios and adjusting the input features accordingly.
Overall, mitigating bias in machine learning models is an important aspect of responsible and ethical AI. By using strategies such as increasing data diversity and using tools such as cross-validation and regularization, developers can create models that are more accurate, fair, and unbiased.
Conclusion
In conclusion, calculating bias is an important step in ensuring the accuracy of research studies and data analysis. Bias can occur in various stages of the data collection and loan payment calculator bankrate analysis process, including the source of the data, the sampling method, and the analytical techniques used.
To calculate bias, one must first identify the errors by subtracting each estimate from the actual or observed value. Then, add up all the errors and divide by the number of estimates to get the bias. If the errors add up to zero, the estimates were unbiased, and the method delivers unbiased results.
It is important to note that bias can have a significant impact on the results of a study, and can even invalidate the conclusions drawn from the data. Therefore, researchers must take steps to minimize bias in their studies, such as using random sampling methods, ensuring the accuracy of data collection instruments, and using appropriate statistical techniques.
By understanding and calculating bias, researchers can ensure that their studies are accurate and reliable, and that the conclusions drawn from the data are valid.
Frequently Asked Questions
What is the formula for calculating bias in statistics?
The formula for calculating bias in statistics is to subtract the true value from the estimated value, then divide the difference by the true value. The resulting quotient is the bias percentage.
How can bias be computed in Excel?
In Excel, bias can be computed by using the AVERAGE function to find the mean of the estimated values, then subtracting the true value from the mean. The resulting difference is the bias.
What are the steps to determine bias in laboratory testing?
To determine bias in laboratory testing, the laboratory must first establish a reference value for the test. Then, the laboratory must test a sample using the same method as the reference value. The difference between the laboratory result and the reference value is the bias.
Can you provide an example of how to calculate bias percentage?
Suppose an estimator is used to estimate the weight of an object. The true weight of the object is 100 grams, but the estimator estimates the weight to be 110 grams. To calculate the bias percentage, subtract the true value from the estimated value, then divide the difference by the true value. In this case, the bias percentage is (110 - 100) / 100 = 0.1 or 10%.
What is the process for finding the bias of an estimator?
The process for finding the bias of an estimator is to compare the expected value of the estimator to the true value of the parameter being estimated. If the expected value is equal to the true value, then the estimator is unbiased. If the expected value is not equal to the true value, then the estimator is biased.
In what way is bias calculated within the context of Machine Learning?
In the context of Machine Learning, bias is calculated by comparing the predicted values to the actual values. If the predicted values consistently overestimate or underestimate the actual values, then the model is biased. The bias can be reduced by adjusting the model parameters or by using a different model.