How to Calculate Expected Value in Chi Square Test: A Clear Guide
Expected value is a key concept used in the Chi Square Test, a statistical test used to determine if there is a significant difference between the expected and observed frequencies in one or more categories. The expected value is the theoretical value that would be obtained if the data followed a certain distribution, and it is used to calculate the Chi Square statistic. Understanding how to calculate expected value is therefore essential for anyone who wants to perform a Chi Square Test.
To calculate the expected value, you need to know the total number of observations and the expected proportion of each category. This can be done by using the formula: expected value = (total number of observations) x (expected proportion). The expected proportion is the proportion of each category that you would expect to see if the data followed a certain distribution. Once you have calculated the expected value for each category, you can use these values to calculate the Chi Square statistic.
The Chi Square Test is commonly used in research to test the independence of two or more variables, such as whether there is a relationship between smoking and lung cancer. By calculating the expected value and comparing it to the observed value, researchers can determine whether the difference between the two is statistically significant, and therefore whether there is evidence of a relationship between the variables. Understanding how to calculate expected value is therefore an important skill for anyone who wants to perform statistical analyses.
Overview of Chi-Square Test
The chi-square test is a statistical test used to determine whether there is a significant association between two categorical variables. It is a non-parametric test, which means it does not rely on any assumptions about the distribution of the data. Instead, it is based on the observed frequencies of the categories in a contingency table.
The test is named after the Greek letter "chi" (χ), which is used to represent the test statistic. The test statistic is calculated by comparing the observed frequencies in each category to the expected frequencies. The expected frequencies are calculated under the assumption that there is no association between the two variables.
The chi-square test is widely used in various fields, including social sciences, biology, and business. It can be used to test hypotheses about the relationship between variables, to identify patterns in data, and to assess the goodness of fit of a model.
To perform a chi-square test, one needs to set up a contingency table with the two categorical variables of interest. The contingency table shows the number of observations in each category. The test statistic is then calculated using a formula that takes into account the observed and expected frequencies. The resulting value is compared to a critical value from a chi-square distribution with a certain number of degrees of freedom. If the test statistic is greater than the critical value, the null hypothesis of no association between the variables is rejected.
In summary, the chi-square test is a powerful tool for analyzing categorical data. It can be used to test hypotheses, identify patterns, and assess model fit. With its wide applicability and ease of use, it is an essential tool for any researcher or analyst working with categorical data.
Understanding Expected Value
Expected value is a term used in statistics to represent the theoretical mean of a probability distribution. In the context of a chi-square test, expected value refers to the number of observations that would be expected in each category if the null hypothesis were true.
To calculate expected value in a chi-square test, you need to know the total number of observations in your sample, as well as the expected proportions for each category under the null hypothesis. Once you have these values, you can use the formula:
Expected value = (row total x column total) / sample size
where the row total is the sum of all observations in a particular row, the column total is the sum of all observations in a particular column, and the sample size is the total number of observations in the sample.
It's important to note that expected values are theoretical values, and may not necessarily match the actual observed values in your sample. However, by comparing the expected values to the observed values, you can determine whether there is a significant difference between the two, and whether this difference is statistically significant.
In summary, expected value is an important concept in chi-square tests, as it allows you to compare the theoretical distribution of observations under the null hypothesis to the actual observed distribution in your sample. By calculating expected values for each category, you can determine whether there is a significant difference between the observed and expected values, and make inferences about the underlying population.
Calculating Expected Value in a Contingency Table
In a chi-square test, the expected value is the value that would be expected in a contingency table if there is no association between the two variables being compared. The expected value can be calculated using the following formula:
Expected value = (row total x column total) / sample size
Identifying Observed Frequencies
Before calculating the expected value, it is necessary to identify the observed frequencies in the contingency table. The observed frequencies are the actual frequencies that are observed in the sample. For example, if the contingency table compares gender and occupation, the observed frequencies would be the number of males and females in each occupation category.
Determining Marginal Totals
To calculate the expected value, it is also necessary to determine the marginal totals for each row and column in the contingency table. The marginal totals are the total number of observations in each row and column. For example, if the contingency table compares gender and occupation, the marginal totals would be the total number of males and females, as well as the total number of individuals in each occupation category.
Once the observed frequencies and marginal totals have been identified, the expected value can be calculated using the formula above. The expected value is then compared to the observed frequency in each cell of the contingency table to determine if there is a significant association between the two variables being compared.
Overall, calculating the expected value in a contingency table is an important step in conducting a chi-square test. By identifying the observed frequencies and determining the marginal totals, researchers can accurately calculate the expected value and determine if there is a significant association between the two variables being compared.
Applying the Chi-Square Formula
Computing Expected Frequencies
To calculate the expected frequencies in a chi-square test, use the formula:
Expected count = (row sum * column sum) / table sum
For example, suppose you have a table with two rows and two columns. The row sums are 200 and 300, while the column sums are 250 and 250. The table sum is 500. To find the expected count for the first row and first column, you would use the following formula:
Expected count = (200 * 250) / 500 = 100
You can repeat this formula for each cell in the table to obtain all the expected counts.
Calculating Chi-Square Statistic
Once you have computed the expected counts, you can proceed to calculate the chi-square statistic. The formula for the chi-square statistic is:
χ² = Σ [(O - E)² / E]
Where:
- Σ is the sum of all the cells in the table
- O is the observed frequency for each cell
- E is the expected frequency for each cell
To calculate the chi-square statistic, follow these steps:
- Compute the difference between the observed and expected frequencies for each cell:
(O - E)
- Square each difference:
(O - E)²
- Divide each squared difference by the expected frequency:
(O - E)² / E
- Sum all the values from step 3:
Σ [(O - E)² / E]
- The result from step 4 is the chi-square statistic.
Once you have calculated the chi-square statistic, you can compare it to a critical value from a chi-square distribution with degrees of freedom equal to (number of rows - 1) * (number of columns - 1)
. If the chi-square statistic is greater than the critical value, you can reject the null hypothesis and conclude that there is a significant association between the two variables being tested.
Interpreting Chi-Square Results
After calculating the expected value in a chi-square test, the next step is to interpret the results. The chi-square test is used to determine if there is a significant association between two categorical variables. The test produces a chi-square statistic and a p-value.
The chi-square statistic measures the difference between the observed and expected values. A higher chi-square statistic indicates a greater difference between the observed and expected values and a stronger association between the two variables. The p-value measures the probability of obtaining a chi-square statistic as extreme or more extreme than the observed value, assuming that there is no association between the two variables.
If the p-value is less than the chosen significance level (usually 0.05), then the null hypothesis is rejected, and there is evidence of a significant association between the two variables. On the other hand, if the p-value is greater than the chosen significance level, then the null hypothesis is not rejected, and there is no evidence of a significant association between the two variables.
It is important to note that a significant association does not imply causation. Therefore, further investigation is required to determine the cause-effect relationship between the two variables.
In summary, interpreting chi-square results involves analyzing the chi-square statistic and the p-value to determine if there is a significant association between two categorical variables. A significant association indicates that the two variables are related, but further investigation is required to determine causation.
Assumptions of the Chi-Square Test
Before performing a Chi-Square Test, it is important to verify that the assumptions of the test are met. The following assumptions are necessary for the Chi-Square Test to be valid:
Both variables are categorical: The first assumption of the Chi-Square Test is that both variables are categorical. This means that the data must be divided into categories or bankrate com calculator groups. For example, the variable could be gender or political affiliation.
Independence: The second assumption is that the observations are independent. This means that the value of one observation does not depend on the value of another observation. For example, if we are studying the relationship between gender and political affiliation, we assume that a person's gender does not influence their political affiliation.
Expected cell count: The third assumption is that the expected cell count for each cell in the contingency table is at least 5. This means that each category or group has a sufficient number of observations to make accurate inferences.
Random Sampling: The fourth assumption is that the data is collected through random sampling. This means that the sample is representative of the population and that the results can be generalized to the population.
If any of these assumptions are violated, the results of the Chi-Square Test may not be valid. Therefore, it is important to carefully consider these assumptions before conducting the test.
Limitations of Chi-Square Test
While the Chi-Square test is a powerful statistical tool, it has some limitations that should be taken into account when interpreting the results.
Sample Size
The Chi-Square test assumes that the sample size is large enough to accurately represent the population. If the sample size is too small, the test may not be reliable. As a general rule, the sample size should be at least 5 for each cell in the table.
Independence Assumption
The Chi-Square test assumes that the observations are independent of each other. If there is any dependence between the observations, the test may produce inaccurate results. For example, if the observations are taken from a time series, there may be autocorrelation between the observations.
Cell Size
The Chi-Square test assumes that the expected frequency for each cell is at least 5. If the expected frequency is less than 5, the test may not be reliable. In this case, Fisher's exact test or Yates' correction for continuity may be used instead.
Interpretation
The Chi-Square test only tells us whether there is a significant association between two variables. It does not tell us the strength or direction of the association. Therefore, it is important to use other statistical measures, such as correlation coefficients, to fully understand the relationship between the variables.
In summary, while the Chi-Square test is a useful tool for analyzing categorical data, it has some limitations that should be taken into account when interpreting the results.
Frequently Asked Questions
What is the formula for calculating expected values in a chi-square test?
The formula for calculating expected values in a chi-square test is:
Expected count = (row sum * column sum) / table sum.
This formula is used to determine the expected frequency for each cell in the contingency table. The expected frequency is then compared to the observed frequency to determine the chi-square statistic.
How can expected frequencies be computed for a chi-square test using a given example?
Expected frequencies can be computed for a chi-square test using a given example by following the formula for expected counts. Once the expected counts are calculated, the chi-square test statistic can be calculated using the formula:
Chi-Square = Σ((O - E)² / E)
where Σ denotes the sum of the calculations for each cell in the contingency table.
In what way does one interpret the results obtained from a chi-square test?
The results obtained from a chi-square test are interpreted by comparing the calculated chi-square statistic to the critical value of the chi-square distribution with the appropriate degrees of freedom and level of significance. If the calculated chi-square statistic is greater than the critical value, then the null hypothesis is rejected, and it is concluded that there is a significant relationship between the variables being tested.
Can you explain the process of determining expected counts for a chi-square test in Excel?
To determine expected counts for a chi-square test in Excel, one can use the formula:
Expected count = (row total * column total) / grand total
This formula can be applied to each cell in the contingency table to calculate the expected counts. The chi-square test statistic can then be calculated using Excel's built-in functions.
What are the steps involved in performing a chi-square test of independence?
The steps involved in performing a chi-square test of independence are as follows:
- State the null and alternative hypotheses.
- Collect the data and organize it into a contingency table.
- Calculate the expected counts for each cell in the contingency table.
- Calculate the chi-square test statistic using the formula: Chi-Square = Σ((O - E)² / E)
- Determine the degrees of freedom and find the critical value of the chi-square distribution.
- Compare the calculated chi-square test statistic to the critical value.
- Interpret the results and draw conclusions.
When is it appropriate to apply the chi-square test in statistical analysis?
The chi-square test is appropriate to apply in statistical analysis when the data being analyzed is categorical or nominal in nature. It is used to determine if there is a significant relationship between two or more variables in a population. It is commonly used in fields such as social sciences, biology, and marketing research.
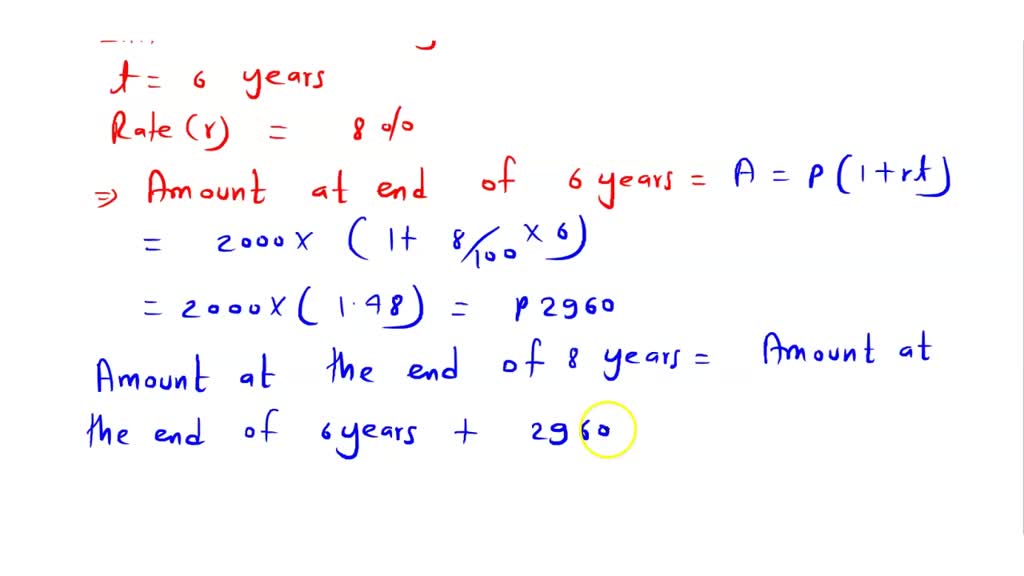