How to Calculate Confidence Limits: A Clear Guide
Calculating confidence limits is an essential part of statistical analysis. Confidence limits are a range of values that are likely to contain the true value of a population parameter. In other words, they provide a measure of uncertainty around a sample statistic, such as the mean or proportion. Confidence limits are commonly used in hypothesis testing, where they are used to determine whether a sample statistic is significantly different from a hypothesized value.
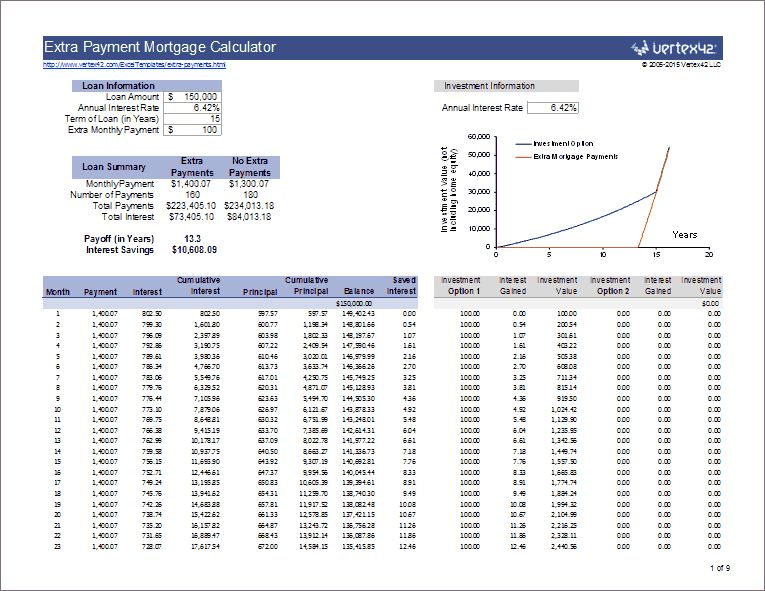
To calculate confidence limits, one needs to know the sample size, the sample statistic, and the level of confidence desired. The level of confidence is typically set at 95%, which means that if the same sample were repeatedly taken from the same population, the calculated confidence interval would contain the true population parameter in 95% of the samples. The confidence level can be adjusted depending on the level of uncertainty allowed.
There are different methods for calculating confidence limits, depending on the type of data and the sample size. For large sample sizes, the normal distribution can be used to calculate confidence limits. For small sample sizes or non-normal data, other methods such as the t-distribution or the bootstrap method may be used. Understanding how to calculate confidence limits is essential for anyone working with statistical data, as it provides a measure of the reliability of the results.
Understanding Confidence Limits
Definition of Confidence Limits
Confidence limits are a statistical concept that refers to the range of values within which a population parameter is expected to lie with a certain degree of confidence. In other words, confidence limits provide a range of plausible values for a population parameter, such as a mean or proportion, based on a sample of data. The level of confidence is typically expressed as a percentage, such as 95% or 99%, and represents the probability that the true population parameter lies within the calculated confidence interval.
To calculate confidence limits, one must first determine the sample size, sample mean, and sample standard deviation. From there, one can use a statistical formula to calculate the confidence interval. The formula takes into account the sample size, the level of confidence, and the standard error of the sample mean.
Significance of Confidence Levels
The level of confidence chosen for calculating confidence limits is an important consideration in statistical analysis. A higher level of confidence, such as 99%, will result in a wider confidence interval and a greater likelihood that the true population parameter lies within the interval. However, a wider interval may be less precise and may not be suitable for certain applications.
Conversely, a lower level of confidence, such as 90%, will result in a narrower confidence interval and a greater precision in estimating the population parameter. However, a narrower interval may be less likely to contain the true population parameter and may not provide sufficient confidence for certain applications.
In summary, understanding confidence limits is essential for statistical analysis and decision-making. By calculating confidence limits with an appropriate level of confidence, researchers and analysts can make informed decisions based on the best available data.
Statistical Background
Central Limit Theorem
The Central Limit Theorem (CLT) is a fundamental concept in statistics that states that as the sample size increases, the distribution of the sample means approaches a normal distribution, regardless of the shape of the population distribution. This theorem is important because it allows us to make inferences about the population mean using sample data.
Standard Error
The standard error (SE) is a measure of the variability of the sample means. It is the standard deviation of the sampling distribution of the mean. The SE is used to calculate confidence intervals and hypothesis tests. A smaller SE indicates less variability in the sample means, and a larger SE indicates more variability.
Z-Scores and T-Scores
Z-scores and t-scores are used to determine the probability of obtaining a particular sample mean given the population mean and standard deviation. Z-scores are used when the population standard deviation is known, and t-scores are used when the population standard deviation is unknown and must be estimated from the sample data.
In summary, understanding the Central Limit Theorem, Standard Error, and Z-scores/T-scores is essential for calculating confidence limits. These concepts allow us to make inferences about the population mean using sample data.
Calculating Confidence Limits
Calculating confidence limits is a crucial step in statistical analysis. Confidence limits provide a range of values that is likely to contain the true population parameter based on the sample data. This section will cover the three main steps in calculating confidence limits: identifying the sample statistics, selecting the confidence level, and using the formula.
Identifying the Sample Statistics
The first step in calculating confidence limits is identifying the sample statistics. These statistics include the sample mean, sample size, and sample standard deviation. The sample mean is the average value of the sample data, the sample size is the number of observations in the sample, and the sample standard deviation is the measure of the spread of the sample data.
Selecting the Confidence Level
The next step is selecting the confidence level. The confidence level is the probability that the true population parameter falls within the calculated confidence interval. The most common confidence level is 95%, but other confidence levels can be used as well.
Using the Formula
The final step is using the formula to calculate the confidence limits. The formula for calculating confidence limits depends on the sample size and the type of distribution of the sample data. For large sample sizes, the normal distribution can be used, while for small sample sizes, the t-distribution should be used.
To calculate the confidence limits for a large sample size with a normal distribution, the formula is:
Confidence Limits = Sample Mean ± (Z Score * Standard Error)
where Z Score is the number of standard deviations from the mean for a given confidence level, and Standard Error is the standard deviation of the sample divided by the square root of the sample size.
For example, if the sample mean is 50, the sample standard deviation is 5, the sample size is 100, and the confidence level is 95%, the Z Score is 1.96. Therefore, the confidence limits would be:
Confidence Limits = 50 ± (1.96 * (5 / sqrt(100)))
Confidence Limits = 48.04 to 51.96
In conclusion, calculating confidence limits involves identifying the sample statistics, selecting the confidence level, and using the appropriate formula. By following these steps, researchers can estimate the range of values that is likely to contain the true population parameter based on the sample data.
Confidence Intervals for Different Distributions
Confidence intervals are used to estimate the range of values within which a population parameter, such as the mean or proportion, is likely to lie. The calculation of confidence intervals depends on the distribution of the sample data. In this section, we will discuss the three most common distributions used in statistical inference: Normal, T-distribution, and other distributions.
Normal Distribution
The Normal distribution is the most commonly used distribution in statistical inference. It is a continuous probability distribution that is symmetric and bell-shaped. When the sample size is large (n -gt; 30), the distribution of the sample mean is approximately Normal, even if the population distribution is not Normal. In this case, the confidence interval can be calculated using the formula:
where x̄ is the sample mean, zα/2 is the critical value of the standard Normal distribution at α/2 level of significance, s is the sample standard deviation, and n is the sample size.
T-Distribution
When the sample size is small (n -lt; 30) and the population standard deviation is unknown, the T-distribution is used to calculate the confidence interval. The T-distribution is a family of distributions that is similar to the Normal distribution but has heavier tails. The critical values of the T-distribution depend on the sample size and the level of significance. The formula for the confidence interval is:
where tα/2 is the critical value of the T-distribution with n-1 degrees of freedom at α/2 level of significance, s is the sample standard deviation, and n is the sample size.
Other Distributions
If the population distribution is not Normal or the sample size is small and the population standard deviation is unknown, other distributions can be used to calculate the confidence interval. For example, if the data are binary (e.g., success or failure), the confidence interval for the proportion can be calculated using the binomial distribution. If the data are counts (e.g., number of defects), the confidence interval for the mean can be calculated using the Poisson distribution. If the data are skewed (e.g., income), the confidence interval can be calculated using the bootstrap method.
In conclusion, the choice of distribution for calculating the confidence interval depends on the nature of the data and the sample size. The Normal distribution is used when the sample size is large, the T-distribution is used when the sample size is small and the population standard deviation is unknown, and other distributions are used when the data are non-Normal or the sample size is small and the population standard deviation is unknown.
Interpreting Confidence Limits
Confidence limits provide a range of values that we estimate a population parameter to lie within. These limits are used to measure the statistical certainty of our results and are crucial in data analysis.
When interpreting confidence limits, it is essential to understand that they do not represent the range of values that the true population parameter can take. Instead, they represent the range of values that we are confident the population parameter lies within.
Confidence limits are calculated based on the sample data and the level of confidence desired. For example, a 95% confidence interval means that if we were to repeat the study multiple times, we would expect the true population parameter to lie within the calculated interval 95% of the time.
It is important to note that the width of the confidence interval is affected by the sample size and the variability of the data. A larger sample size or lower variability will result in a narrower confidence interval, indicating greater precision in our estimate of the population parameter.
When interpreting confidence limits, it is crucial to avoid common misconceptions that can lead to misinterpretation of results. For example, it is incorrect to assume that the true population parameter lies within the calculated interval with a probability of 95%. Instead, the interval either contains the true population parameter or it does not.
In conclusion, interpreting confidence limits requires a nuanced understanding of their role in data analysis. Confidence limits provide a measure of statistical certainty, and their interpretation should be based on the level of confidence desired and the variability of the data.
Common Misconceptions
Confidence intervals are widely used in research and data analysis to estimate population parameters. However, there are some common misconceptions that can lead to misinterpretation of results. Here are some of the most common misconceptions about confidence intervals:
Misconception 1: The Confidence Interval Represents the Range of Values in the Population
One common misconception is that the confidence interval represents the range of values in the population. However, this is not true. The confidence interval is a range of values that is likely to contain the population parameter based on the sample data. The population parameter is the true value of the parameter in the population, and it is usually unknown.
Misconception 2: A 95% Confidence Interval Means that There is a 95% Probability that the True Value Lies within the Interval
Another common misconception is that a 95% confidence interval means that there is a 95% probability that the true value lies within the interval. However, this is not true either. The confidence level of a confidence interval is the probability that the interval will contain the true value of the parameter in repeated sampling. It is not the probability that the true value lies within the interval in a single sample.
Misconception 3: The Confidence Interval Tells Us How Precise the Estimate Is
A third common misconception is that the confidence interval tells us how precise the estimate is. However, this is not entirely true. The width of the confidence interval does give us an idea of how precise the estimate is, but it also depends on the sample size and the variability of the data. A wider interval does not necessarily mean that the estimate is less precise.
Misconception 4: The Confidence Interval Tells Us Whether the Parameter is Statistically Significant
Finally, some people think that the confidence interval tells us whether the parameter is statistically significant. However, this is not true either. Statistical significance is determined by comparing the confidence interval to a null hypothesis value. The confidence interval alone cannot tell us whether the parameter is statistically significant or not.
Overall, it is important to have a clear understanding of what confidence intervals represent and what they do not represent. By avoiding these common misconceptions, researchers and analysts can ensure that their results are interpreted correctly.
Applications of Confidence Limits
Scientific Research
Confidence limits are widely used in scientific research to estimate the population parameter with a certain degree of confidence. Researchers use confidence limits to determine whether their results are statistically significant or not. For example, if a researcher wants to determine the mean weight of a certain species of fish in a lake, they can take a sample of fish and calculate the mean weight. By using confidence limits, the researcher can estimate the range of values within which the true population mean weight is likely to lie. This can help the researcher determine whether the difference between the sample mean and the hypothesized population mean is statistically significant or not.
Business and Economics
Confidence limits are also used in business and economics to estimate parameters such as demand, sales, and profits. For example, a company may use confidence limits to estimate the demand for a new product. By using confidence limits, the company can estimate the range of values within which the true demand is likely to lie. This can help the company determine the optimal price point for the product and the expected profitability.
Engineering and Quality Control
Confidence limits are used in engineering and quality control to estimate parameters such as the mean and standard deviation of a product or process. For example, a manufacturer may use confidence limits to estimate the mean diameter of a certain type of bolt. By using confidence limits, the manufacturer can estimate the range of values within which the true mean diameter is likely to lie. This can help the manufacturer determine whether the product meets the required specifications and quality standards.
In summary, confidence limits are a powerful statistical tool that is widely used in various fields such as scientific research, business and economics, and engineering and quality control. By using confidence limits, researchers and practitioners can estimate the range of values within which the true population parameter is likely to lie, and make informed decisions based on the statistical significance of their results.
Frequently Asked Questions
What is the process for calculating a 95% confidence interval for a given dataset?
To calculate a 95% confidence interval for a given dataset, you need to determine the sample mean, sample standard deviation, and sample size. Once you have these values, you can use the formula to calculate the confidence interval. The formula is:
Confidence Interval = Sample Mean ± (Critical Value × Standard Error)
The critical value depends on the confidence level and the sample size. You can look up the critical value in a statistical table or use a calculator to find it. The standard error is the standard deviation of the sample divided by the square root of the sample size.
How can one use Excel to determine the confidence interval for a set of values?
To determine the confidence interval for a set of values in Excel, you can use the CONFIDENCE function. The syntax of the function is:
CONFIDENCE(alpha, standard_dev, size)
where alpha is the significance level, standard_dev is the standard deviation of the sample, and size is the sample size. The function returns the confidence interval for the mean of the sample. You can also use the CONFIDENCE.NORM function or the CONFIDENCE.T function to calculate the confidence interval using the normal distribution or the t-distribution, respectively.
What is the formula to find the upper and lower limits of a confidence interval?
The formula to find the upper and lower limits of a confidence interval is:
Lower Limit = Sample Mean - (Critical Value × Standard Error)
Upper Limit = Sample Mean + (Critical Value × Standard Error)
where the critical value depends on the confidence level and the sample size, and the standard error is the standard deviation of the sample divided by the square root of the sample size.
How is the confidence limit applied in analytical chemistry?
In analytical chemistry, the confidence limit is used to estimate the precision and accuracy of a measurement. The confidence limit is calculated based on the standard deviation of the measurements and the number of replicates. The confidence limit can be used to determine if a measurement is within the acceptable range of values or if it is significantly different from the expected value.
What method is used to calculate the lower confidence limit for a sample mean?
The method used to calculate the lower confidence limit for a sample mean depends on the distribution of the data. If the data follows a normal distribution, the lower confidence limit can be calculated using the z-score. If the data does not follow a normal distribution, the lower confidence limit can be calculated using the t-score.
How is the z-score used to compute a 95% confidence interval?
The z-score is used to compute a 95% confidence interval when the data follows a normal distribution. The z-score represents the number of standard deviations from the mean. To calculate the z-score, you subtract the sample mean from the population mean and divide by the standard deviation of the population. Once you have the z-score, you can use it to calculate the confidence interval using the formula:
Confidence Interval = Sample Mean ± (Z-Score × Standard Error)
where the standard error is the standard deviation of the sample divided by the square root of the sample size.