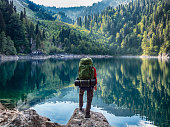
How to Calculate the CDF: A Clear and Confident Guide
The cumulative distribution function (CDF) is a concept used in probability theory to describe the probability distribution of a random variable. The CDF is a function that maps a real number to the probability that a random variable is less than or equal to that number. It is a fundamental concept in probability theory and is used in many applications, including finance, engineering, and physics.
Calculating the CDF can be a complex task, particularly for continuous random variables. However, there are several techniques that can be used to simplify the process. These include using the probability density function (PDF) of the random variable, using the properties of the CDF, and using numerical integration methods. By understanding the basic principles of the CDF and the techniques used to calculate it, it is possible to gain a deeper understanding of probability theory and its applications.
Understanding Cumulative Distribution Functions
A Cumulative Distribution Function (CDF) is a probability distribution function that gives the probability that a random variable X is less than or equal to a specific value x. The CDF is a non-decreasing function that ranges from 0 to 1. It is a useful tool for understanding the probability distribution of a random variable.
The CDF is defined as:
CDF(x) = P(X ≤ x)
>Where X is the random variable, and x is a specific value. The CDF gives us the probability that the random variable X is less than or equal to x. The CDF can be used to calculate the probability of a range of values of X.
>The CDF is a cumulative function because it sums the total likelihood up to that point. The CDF is always non-decreasing, meaning that its value cannot decrease as x increases. The CDF can be used to calculate the probability of a range of values of X.
>The CDF can be graphed to show the probability distribution of a random variable. The graph of the CDF is a step function, with the value of the CDF increasing at each step. The CDF can be used to calculate the probability of a range of values of X.
>In summary, the CDF is a useful tool for understanding the probability distribution of a random variable. The CDF gives us the probability that the random variable X is less than or equal to a specific value x. The CDF is always non-decreasing and can be used to calculate the probability of a range of values of X.
Prerequisites for Calculating CDFs
>>
Probability Theory Basics
>Before diving into the calculation of Cumulative Distribution Functions (CDFs), it is essential to understand some basics of probability theory. Probability theory is the branch of mathematics that deals with the analysis of random phenomena. It is used to model and analyze situations where the outcome is uncertain.
>The fundamental concept of probability theory is the probability of an event. The probability of an event is a number between 0 and 1, which represents the likelihood of the event occurring. A probability of 0 means that the event is impossible, and a probability of 1 means that the event is certain.
>Random Variables and Probability Distributions
>A random variable is a variable whose value is determined by the outcome of a random event. For example, the result of a coin toss can be represented as a random variable, where the value of the variable is either "heads" or "tails."
>A probability distribution is a function that describes the likelihood of the possible values of a random variable. There are two types of random variables: discrete and continuous.
>Discrete vs. Continuous Variables
>Discrete random variables can only take on a finite or countably infinite number of values. Examples of discrete random variables include the number of heads in a series of coin tosses or the number of cars sold in a day.
>Continuous random variables can take on any value within a range of values. Examples of continuous random variables include the height of a person or the time it takes to complete a task.
>In order to calculate the CDF of a random variable, one must first determine the probability distribution of the variable. For discrete random variables, the probability distribution can be represented as a probability mass function (PMF). For continuous random variables, the probability distribution can be represented as a probability density function (PDF).
>Understanding the basics of probability theory, random variables, and probability distributions is essential for calculating CDFs. By utilizing these concepts, one can accurately determine the probability of a random variable being less than or equal to a specific value.
Steps to Calculate the CDF for Discrete Random Variables
>>
Identify the Probability Mass Function
>The first step in calculating the CDF for bankrate piti calculator a discrete random variable is to identify its probability mass function (PMF). The PMF gives the probability that the random variable takes on a particular value. It is usually denoted by f(x), where x is the value that the random variable can take on.
>Once the PMF is identified, it is important to make sure that it satisfies the following two properties:
>>f(x) is always greater than or equal to zero.>The sum of f(x) over all possible values of x is equal to one.>>Summing Probabilities Incrementally
>The second step in calculating the CDF for a discrete random variable is to sum the probabilities incrementally. The CDF is defined as the probability that the random variable takes on a value less than or equal to a given value. Mathematically, it can be expressed as follows:
>F(x) = P(X ≤ x)
/>
To calculate the CDF, start by summing the probabilities of all possible values of the random variable that are less than or equal to x. This can be done incrementally by adding up the probabilities one by one. For example, if x = 3, then the CDF can be calculated as follows:
/>
F(3) = P(X ≤ 3) = f(0) + f(1) + f(2) + f(3)<
/>
It is important to note that the CDF is a cumulative function, which means that it increases monotonically as x increases. In other words, as x increases, the probability that the random variable takes on a value less than or equal to x also increases.<
/>
In summary, calculating the CDF for a discrete random variable involves identifying its PMF and summing the probabilities incrementally. By following these steps, one can accurately calculate the probability that the random variable takes on a value less than or equal to a given value.
Steps to Calculate the CDF for Continuous Random Variables
/>
<
/>
Determine the Probability Density Function
/>
The first step in calculating the CDF for continuous random variables is to determine the probability density function (PDF). The PDF is a function that describes the probability of a random variable taking on a certain value. It is defined as the derivative of the cumulative distribution function (CDF).<
/>
There are many different types of probability density functions, each with their own unique characteristics. Some common examples include the normal distribution, the exponential distribution, and the uniform distribution. To determine the PDF for a given continuous random variable, it is important to understand the characteristics of the distribution and how they relate to the variable.<
/>
Integrate the PDF Over the Range
/>
Once the PDF has been determined, the next step is to integrate it over the range of values for which the CDF is being calculated. This involves finding the area under the curve of the PDF. The integral of the PDF gives the cumulative distribution function (CDF) for the random variable.<
/>
To integrate the PDF, it is often necessary to use calculus. The integral can be evaluated using integration by substitution, integration by parts, or other integration techniques. It is important to use the appropriate integration technique for the given PDF and range of values.<
/>
In summary, calculating the CDF for continuous random variables involves determining the probability density function and integrating it over the range of values. This process can be complex and may require the use of calculus. However, with a clear understanding of the characteristics of the distribution and the appropriate integration techniques, it is possible to accurately calculate the CDF for a given continuous random variable.
Interpreting CDF Graphs
/>
<
/>
A CDF graph is a visual representation of the cumulative distribution function, which shows the probability distribution of a random variable. The x-axis of the graph represents the possible values of the random variable, while the y-axis represents the cumulative probability of the variable taking on a value less than or equal to the corresponding x-value.<
/>
Interpreting a CDF graph involves understanding the shape of the curve and the information it conveys about the probability distribution. The following are some key points to keep in mind:<
/><
/><
/>
The CDF curve always starts at 0 on the y-axis and ends at 1 on the y-axis, since the probability of any value less than the minimum possible value is 0, and the probability of any value greater than the maximum possible value is 1.<
/>
/><
/>
A steeper slope on the CDF curve indicates a higher probability density function (PDF) for the corresponding x-value. In other words, values with a steeper slope have a higher likelihood of occurring.<
/>
/><
/>
The inflection points on the CDF curve indicate where the PDF transitions from concave up to concave down or vice versa. These points can be used to identify the mean and median of the distribution.<
/>
/><
/>
The area under the CDF curve between two x-values represents the probability of the random variable taking on a value within that range. This can be calculated by subtracting the CDF value at the lower x-value from the CDF value at the higher x-value.<
/>
/>
/>
Overall, interpreting CDF graphs is an important skill for understanding probability distributions and making informed decisions based on data analysis.
Common Distributions and Their CDFs
/>
Normal Distribution
/>
The normal distribution, also known as the Gaussian distribution, is one of the most commonly encountered probability distributions. It is characterized by a bell-shaped curve and is often used to model real-world phenomena such as heights, weights, and IQ scores. The normal distribution has two parameters, the mean and the standard deviation, which determine the shape of the curve.<
/>
The CDF of the normal distribution cannot be expressed in a closed-form equation, but it can be approximated using numerical methods. However, tables and software are widely available that can provide accurate values of the CDF for any given mean and standard deviation.<
/>
Uniform Distribution
/>
The uniform distribution is a probability distribution in which all values between two endpoints are equally likely. It is often used to model situations where all outcomes are equally likely, such as rolling a fair die or picking a card from a shuffled deck. The uniform distribution has two parameters, the lower and upper endpoints, which determine the range of possible values.<
/>
The CDF of the uniform distribution is a simple linear function that increases uniformly from 0 to 1 as the value of the random variable increases from the lower endpoint to the upper endpoint.<
/>
Exponential Distribution
/>
The exponential distribution is a probability distribution that models the time between events in a Poisson process. It is often used to model the lifetimes of electronic components, the time between arrivals of customers at a service center, or the time between radioactive decay events. The exponential distribution has one parameter, the rate parameter, which determines the rate at which events occur.<
/>
The CDF of the exponential distribution is a simple exponential function that decreases from 1 to 0 as the value of the random variable increases from 0 to infinity.<
/>
In summary, the CDFs of the normal, uniform, and exponential distributions are well-known and widely used in various fields of study. Knowing how to calculate the CDF of these distributions is essential for understanding and analyzing data in many real-world applications.
Numerical Methods for CDF Calculation
/>
Using Computational Tools
/>
Numerical methods for calculating the CDF are often used in situations where analytical solutions are not available or are too complex to compute. Computational tools such as Python, R, and MATLAB have built-in functions for calculating the CDF of various probability distributions. These functions use numerical integration techniques to approximate the CDF.<
/>
For example, in Python, the scipy.stats
module provides functions for calculating the CDF of various probability distributions. The cdf()
function can be used to calculate the CDF of a given distribution at a specified point. The ppf()
function can be used to calculate the inverse CDF, also known as the quantile function.<
/>
Approximation Techniques
/>
When analytical or computational methods are not feasible, approximation techniques can be used to estimate the CDF. One such technique is Monte Carlo simulation, which involves generating random samples from the distribution and using these samples to estimate the CDF.<
/>
Another technique is numerical integration, which involves dividing the range of the distribution into small intervals and approximating the area under the density curve within each interval using numerical methods such as the trapezoidal rule or Simpson's rule.<
/>
Approximation techniques can also be used to approximate the CDF of a distribution for which no closed-form expression is available. One such technique is the Edgeworth expansion, which involves approximating the CDF using a series expansion of the density function. Another technique is the saddlepoint approximation, which involves approximating the CDF using the saddlepoint of the density function.<
/>
Overall, numerical methods and approximation techniques provide useful tools for calculating the CDF in situations where analytical or computational methods are not feasible or are too complex to compute.
Applications of CDFs in Statistical Analysis
/>
Cumulative Distribution Functions (CDFs) are widely used in statistical analysis to calculate probabilities and make predictions based on sample data. Here are some of the most common applications of CDFs in statistical analysis:<
/>
1. Hypothesis Testing
/>
CDFs are used to test hypotheses about the population mean, variance, and other parameters. The CDF of a sample is compared to the CDF of a known distribution to determine the probability that the sample came from that distribution.<
/>
2. Confidence Intervals
/>
CDFs are used to calculate confidence intervals for population parameters. The area under the CDF between two values represents the probability that the population parameter falls within that range.<
/>
3. Survival Analysis
/>
CDFs are used in survival analysis to estimate the probability that an event will occur at or before a given time. The survival function is the complement of the CDF and represents the probability that the event has not occurred by a given time.<
/>
4. Risk Analysis
/>
CDFs are used in risk analysis to estimate the probability of a particular outcome or event occurring. The CDF can be used to calculate the expected value, variance, and other statistical measures of risk.<
/>
Overall, CDFs are a powerful tool in statistical analysis that can be used to make predictions, test hypotheses, and estimate probabilities. By understanding the applications of CDFs, statisticians can make more accurate and informed decisions based on sample data.
Frequently Asked Questions
/>
What is the process for determining a cumulative distribution function from a data table?
/>
To determine a cumulative distribution function (CDF) from a data table, one must first sort the data in ascending order and calculate the probability of each value occurring. Next, the cumulative probability of each value is calculated by adding the probabilities of all the values that are less than or equal to the current value. The resulting values are plotted against the corresponding data values to create a CDF graph.<
/>
What steps are involved in finding the cumulative distribution function in statistical analysis?
/>
The steps involved in finding the cumulative distribution function (CDF) in statistical analysis depend on the type of data being analyzed. For continuous data, the CDF can be determined by integrating the probability density function (PDF) over the range of interest. For discrete data, the CDF can be found by summing the probabilities of all the values up to and including the current value.<
/>
How can one convert a probability density function (PDF) into a cumulative distribution function (CDF)?
/>
To convert a probability density function (PDF) into a cumulative distribution function (CDF), one must integrate the PDF over the range of interest. The resulting integral is the CDF.<
/>
Can you explain the method to derive a CDF from a probability mass function (PMF)?
/>
To derive a CDF from a probability mass function (PMF), one must sum the probabilities of all the values up to and including the current value. The resulting values are plotted against the corresponding data values to create a CDF graph.<
/>
What is the approach to compute the cumulative distribution function for a normal distribution?
/>
The approach to compute the cumulative distribution function (CDF) for a normal distribution involves using the standard normal distribution table or a statistical software package. The CDF can be calculated by finding the area under the normal curve to the left of a given value.<
/>
How does one interpret a cumulative distribution function graph in probability theory?
/>
In probability theory, a cumulative distribution function (CDF) graph shows the probability of a random variable being less than or equal to a given value. The graph is a step function that starts at zero and increases to one. The steepness of the curve indicates the probability density of the data. The CDF can be used to calculate the probability of a value falling within a certain range.